Truveta Data
Truveta Language Model
Clinically trained AI accurately cleaning billions of EHR datapoints daily
Healthcare organizations generate vast amounts of data, yet 95% goes unused – largely because it is fragmented, inaccessible, and unstructured
Truveta Language Model is a large-language, multi-modal AI model that cleans billions of daily EHR data points with industry-leading precision, enabling scientifically rigorous research.
Daily updated EHR data cleaned with AI

TLM’s healthcare expertise is trained on the largest collection of complete medical records for more than 100 million patients, representing the full diversity of the United States.

TLM can clean all types of EHR data, including semi-structured data such as lab tests or diagnoses, or unstructured data such as the contents of clinical notes or imaging reports.
Raw medical text is normalized to the most appropriate medical ontology with standard units of measurement, ensuring research-ready inputs.
TLM maps clinical concepts to standard medical ontologies
For example, TLM’s data cleaning process is applied to lab test results below. TLM structured two sets of lab test results into four rows of the LabResult table within the Truveta Data Model (TDM). Each test is mapped to a standard medical ontology with standard units of measurement.
TLM mapping lab results to the appropriate standard medical ontology
Accelerate access to data hidden in notes
Example data extracted from a cardiac catheterization report.



Conduct research confidently with high-quality data



Unlike general large language models, which understand language but are often inaccurate within the medical domain due to being trained on the public Internet, TLM’s specialized training on healthcare data is critical for ensuring the clinical validity of Truveta Data.
TLM normalization capabilities compared to human experts
TLM normalization capabilities compared to human experts



Unlike general large language models, which understand language but are often inaccurate within the medical domain due to being trained on the public Internet, TLM’s specialized training on healthcare data is critical for ensuring the clinical validity of Truveta Data.
Learn more about the depth of Truveta Data
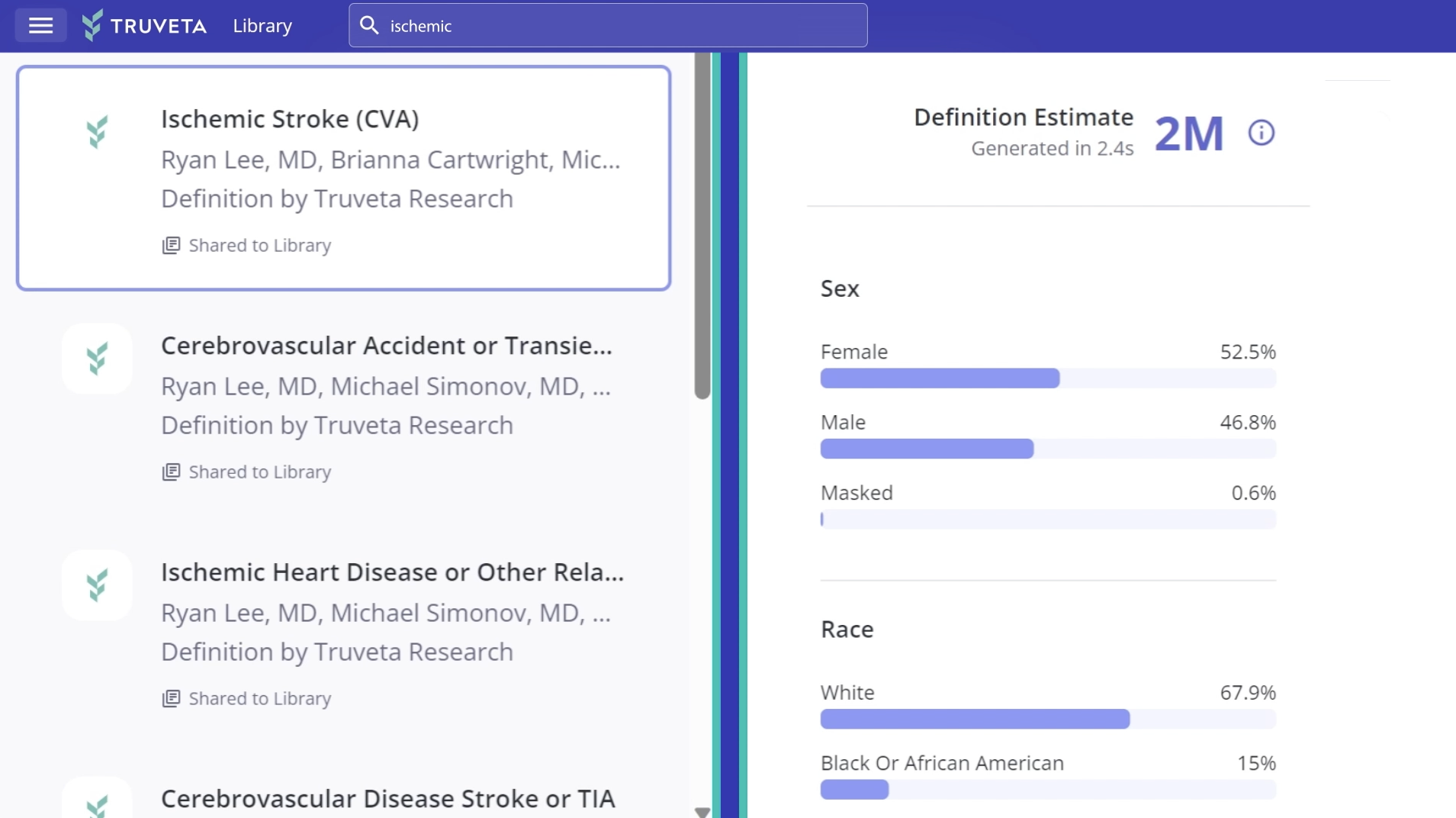
Complete and clean EHR data
Truveta offers complete, timely, and clean EHR data linked with SDOH, mortality, and claims data for more than 120M patients representing the full diversity of the US.
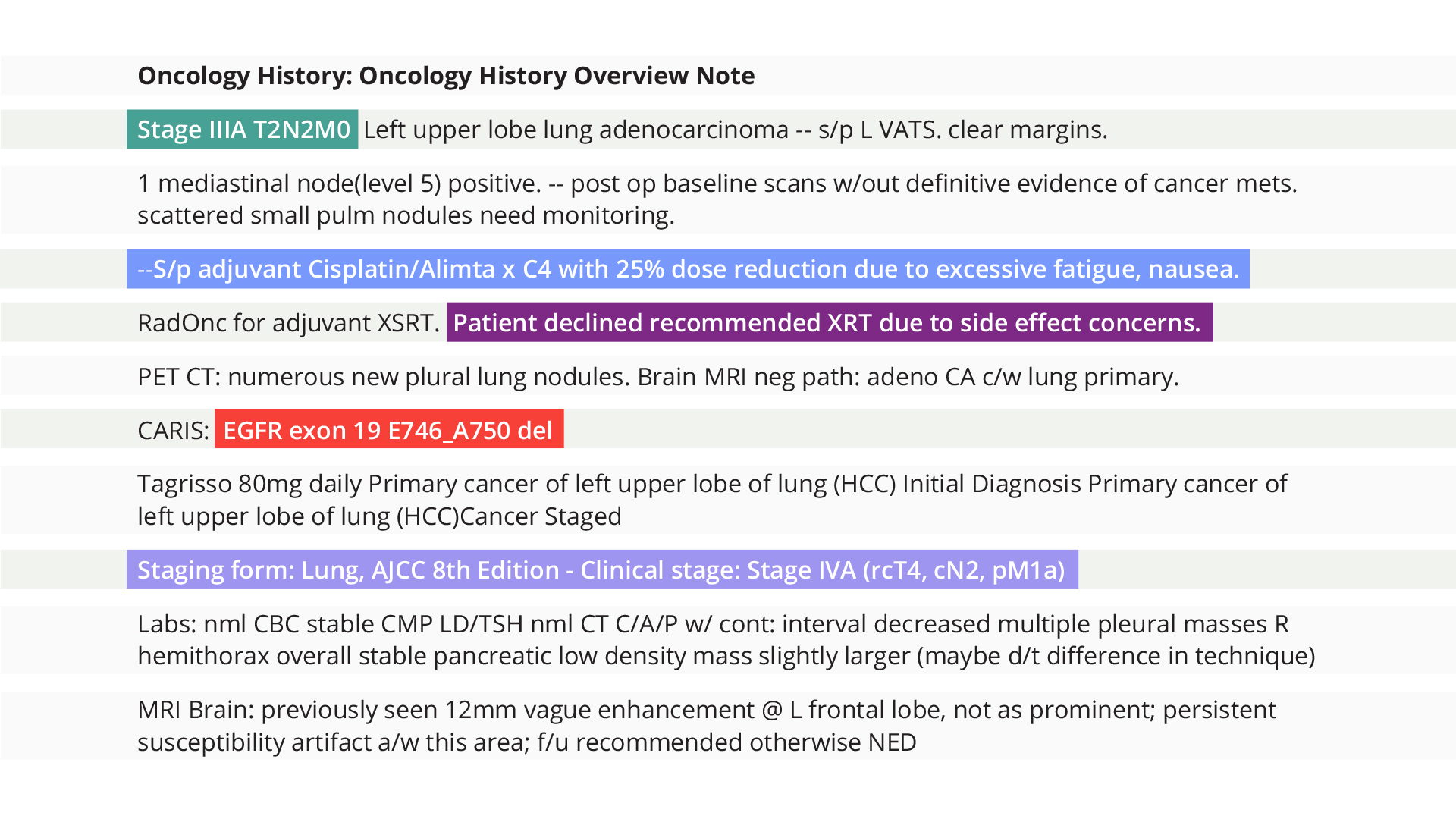
More than 5 billion clinical notes
The Truveta Language Model cleans and structures data from notes at scale, enabling researchers to understand patients’ complete clinical context and answer novel research questions.